Introduction
Artificial intelligence (AI) amalgamates diverse intelligent processes and behaviors, harnessed through computational models, algorithms, or rule sets, enabling machines to emulate human cognitive functions like learning and problem-solving. Rapidly permeating the healthcare sector, AI significantly influences clinical decision-making, disease diagnosis, and automation. The potential for AI to further explore pharmaceutical and healthcare research is immense, driven by its capacity to analyze vast datasets from various modalities.1
Within healthcare, AI technologies encompass machine learning (ML), natural language processing (NLP), physical robots, and robotic process automation. ML, especially neural network models and deep learning, is applied to imaging data for early identification of clinically significant elements, particularly in cancer-related diagnoses. NLP employs computational techniques to comprehend human speech and derive meaning, increasingly incorporating ML for exploring unstructured data in doctors' notes, lab reports, etc., aiding decision-making in diagnosis and treatment options.2 These innovations pave the way for precise and rapid diagnoses and customized treatment interventions, enhancing patient outcomes.
AI-based solutions leverage diverse data types, including patient-reported symptoms, biometrics, imaging, and biomarkers. Advancements in AI enable early detection of potential illnesses, significantly improving the chances of prevention.3 Physical robots find applications in various healthcare segments, from nursing and telemedicine to cleaning, radiology, surgery, and rehabilitation. Robotic process automation, an inexpensive and easily programmable technology, streamlines structured digital tasks for administrative purposes within the healthcare system. 4
In the pharmaceutical sector, AI plays a pivotal role across various stages, from drug discovery to product management. AI technologies, such as ML, deep learning, and AI-based quantitative structure-activity relationship (QSAR) technologies, are integral in drug screening and design. Neural networks, including artificial neural networks (ANNs), deep neural networks (DNNs), and recurrent neural networks (RNNs), facilitate data processing in drug development. SVMs contribute to classification and regression of input data. In pharmaceutical manufacturing, AI ensures automated and personalized manufacturing, error matching, and quality control. 5
AI's incorporation in clinical trials aids subject selection, reduces dropouts through close monitoring, and employs ML for various aspects. Furthermore, AI technologies, like as ML and NLP tools, are critical in market analysis, product positioning, and price. Recent papers highlight AI's use in medicinal chemistry, healthcare, pharmaceutical, and biomedical research, with an emphasis on target protein discovery, computer-aided drug design, virtual screening, and illness detection, notably in cancer. This review excludes studies unrelated to healthcare/pharmaceutical research, ensuring a focused exploration of AI's impact on disease diagnosis, drug discovery, clinical trials, personalized treatment, and epidemiological research. The search strategy involves specific keywords across PubMed, Science Direct, and Google Scholar domains. 6, 7
AI Tool Application in Dosage form Designs
To understand the influence of medication distribution, the human body system is separated into compartments, with biological membranes playing a critical role in this process. The rate of permeation determined by the route of administration is a key indicator for monitoring an efficient drug delivery system. Passive diffusion relies on the chemical characteristics of the medication, whereas active penetration is triggered by membrane transport and largely depends on complicated biological interactions. 8
To investigate the pharmacokinetic characteristics of the drug delivery system, a more recent computer model is applied. The predictability of preclinical models based on specified parameters is a critical need in the pharmaceutical industry's research and development. AI can give advanced technology for analysing multilayer data, resulting in a better comprehension of research units. 9 AI may be utilised to do pharmacokinetic research utilising innovative AI technologies such as artificial neural networks, as well as to offer databases such as chemical, genomic, and phenotypic databases for a better understanding of drug interaction and the complicated unit functions of molecules. Some approaches are also used to investigate the influence of the drug delivery mechanism on the pharmacokinetics of the medication, allowing for a more complete knowledge of disposition and toxicity. 10, 11
AI can help with drug development and drug repurposing strategies, which include applying current therapies to new ailments. Patients' needs and medical circumstances are important variables in formulation, pharmacokinetics, and medication development. The availability of precise information databases is a key difficulty linked with the full-scale deployment of AI to construct delivery systems. 12 By leveraging existing information, processing or digesting vast volumes of data, and executing more vigorous codification inside the knowledge database with outstanding self-supervised experimentation outcomes and connected to accurate parameter recording, AI aids future applications. In conclusion, AI plays a vital role in drug delivery, addressing the challenges of predicting drug distribution, understanding drug interactions, and developing effective drug delivery systems. By utilizing AI tools and databases, we can improve the efficiency and effectiveness of drug delivery systems in various clinical settings. 13
AI for Drug Delivery
The use of AI and big data in the pharmaceutical business has resulted in the establishment of computational pharmaceutics, which seeks to improve drug delivery systems via the use of multiscale modelling methodologies. AI algorithms and machine learning techniques are used in computational pharmaceutics to analyse massive datasets and anticipate drug behaviour, allowing researchers to assess numerous scenarios and optimise drug delivery systems without the need for laborious trial-and-error studies. 14 This shortens the medication development timeframe, lowers expenses, and boosts productivity. By utilising computational pharmaceutics, researchers can also gain insights into the interactions between drugs and the human body, leading to more personalised and effective treatment options. Additionally, the integration of AI algorithms in drug delivery systems can help identify potential side effects or drug-drug interactions, ensuring patient safety and reducing the risk of adverse reactions. 15, 16
AI algorithms may analyse complicated interactions between drug characteristics, formulation components, and physiological parameters to anticipate drug behaviour at several scales, providing for a more complete knowledge of drug delivery processes and assisting in the design of effective drug delivery systems. It aids in the prediction of the medication's physicochemical qualities, in vitro drug release profile, and drug stability.17 The same method is also used to improve in vivo pharmacokinetic parameters and medication distribution, as well as in vivo-in vitro correlation research. Researchers may discover possible risks and obstacles connected with drug delivery systems early in the development process by using the correct combination of AI tools, allowing for proactive tweaks and adjustments to limit risks and optimise drug delivery systems. 18
AI for oral solid dosage form development
s are one of the most used solid dose forms in the pharmaceutical business. AI can aid in the search for optimum formulations and the investigation of desirable process characteristics. To produce dependable dosage forms and get a better knowledge of the inputs and outputs for processing and operations, artificial neuralnetworks (ANNs), fuzzy logics, neural networks, and evolutionary algorithms are employed. 19, 20 Tablets are a popular solid dosage form, accounting for a sizable percentage of the medication delivery industry. This product is made by combining active pharmaceutical ingredients and excipients, which are subsequently crushed or moulded to get the required shape and size. Excipients are substances that are added to tablets in order to influence the intended product outcome, such as tablet disintegration, dissolving, and medicine release. AI may also be used in the context of systemic drug administration to forecast drug release and examine the implications of critical tablet production factors, with the ability to assure consistent quality control procedures. 21 Certain AI programmes have been used to detect flaws in tablets. 22
The ability to predict dug release using formulations offers the potential to provide reliable quality control. Drug release studies are conducted using in vivo and in vitro methodologies, which are regarded as core technologies that are examined or tested on a regular basis during product development. AI is used in medication formulation to help forecast drug release, resulting in fewer runs required to optimise the batch, decreasing effort and expense during pilot batch scale and production processes.21, 23 AI may aid in the prediction of drug release and dissolution profiles, as well as the exploration of disintegration time for the effective selection of the best batch for subsequent scale processing. 24
AI for nanomedicine
Using artificial intelligence (AI) skills in data analysis, pattern recognition, and optimisation offers great potential for nanomedicine researchers. This integration has the potential to accelerate the discovery of novel nanoscale therapies, improve diagnostics, improve medication delivery, and advance personalised medicine. The potential influence of AI in nanomedicine includes the ability to provide precise and focused treatment techniques at the nanoscale, which has the potential to revolutionise healthcare. 25, 26 Nanoparticles are important in medicine delivery, AI algorithms help researchers create and optimise nanoparticles for specific applications by predicting their physicochemical qualities, stability, and efficacy. 27 AI-driven inputs such as medication selection, dosage determination, and the selection of stimuli-responsive materials are required for the effective use of nanomedicines as drug delivery carriers, notably in the treatment of cancer patients. Particularly, deep learning algorithms used to treat melanoma have shown great accuracy in patient care and diagnostic processes. 28 AI algorithms are also important in simulating the behaviour and interactions of nanoscale materials inside biological systems. 29 This modelling capability allows for predictions concerning nanoparticle behaviour, drug release kinetics, and potential toxicity, enabling the development of safe and effective nanomedicine formulations. 30 Furthermore, AI is used in nanosensors and biosensors to monitor biomarkers, medication levels, and illness development in real time. These sensors give healthcare practitioners with continuous input, allowing for rapid interventions and personalised therapy modifications. 31
The incorporation of AI-based databases is beneficial for scaling up nanocarriers via automated methods. AI is used in nanocarrier drug delivery systems to optimise nanocarriers and undertake medication compatibility testing using computational methodologies. These methodologies evaluate drug loading, formulation stability, and drug retention, with the goal of ultimately improving therapeutic nanocarriers for specific cell types in tumour therapy. 32 Machine learning approaches are used by researchers such as Yuan He et al. to anticipate nanocrystals made by high-pressure homogenization and wet ball milling, eliminating the requirement for experiment repetition using computational techniques such as Monte Carlo simulations and molecular dynamics. 33 In cooperation with structural nanobiology, including AI in the creation of database repositories for nanocarriers assists in defining 3D structures and exploring physical and chemical characteristics. These repositories are critical for investigating the link between nanocarrier structure and toxicological, physical, and biological data. 34
Lutz Nuhn's research demonstrates the use of AI in analysing the heterogeneous vascular permeability of nanoparticle-based medication delivery systems. These findings will help in the development of protein nanoparticle drug delivery systems with active transendothelial permeability into tumours.35 Similarly, Zhoumeng Lin et al. use AI in conjunction with a physiologically based pharmacokinetic (PBPK) modelling technique to research cancer medication, offering a deeper knowledge of the variables causing low nanoparticle tumour delivery effectiveness. 36
Applicability of AI in parenteral, transdermal, and mucosal routes
Artificial intelligence (AI) plays a pivotal role in the development and manufacturing of complex pharmaceutical formulations, including injectable and biologics. AI systems aid in predicting physicochemical parameters for drug formulations, optimizing pH, solubility, stability, and viscosity by analyzing formulation components and manufacturing processes. This results in the creation of stable parenteral formulations with enhanced quality, efficiency, and variability. 27, 37
AI algorithms play a crucial role in optimizing the production of parenteral products by uncovering the key process factors that impact product qualities. The real-time analysis of process data enhances the uniformity of products, minimizes batch failures, and boosts overall manufacturing productivity. By scrutinizing extensive datasets derived from analytical tests like particle size analysis and chromatography, AI identifies patterns and variations. This enables the early detection and resolution of quality concerns, ensuring a more efficient and reliable production process. In the context of visual inspection, AI is employed to analyze particle behavior within containers, differentiating between swimming, sinking, and sticking particles. Techniques like particle tracking and deep learning algorithms are utilized for accurate inspection and isolation of particles. 16, 38 AI-based image processing is also applied to address issues related to parenteral batch flaws, particularly bubble formation. AI extends its impact to maintenance procedures for complex manufacturing equipment. AI algorithms foresee equipment breakdowns and plan proactive maintenance by analysing sensor data, equipment performance history, and maintenance records. 39 This not only eliminates downtime but also increases productivity and lowers maintenance expenses. AI also assures regulatory compliance in the manufacture of parenteral and complicated biological products. 27 Based on process data and product attributes, AI algorithms analyse compliance, detect possible noncompliance issues, and recommend process improvements. This contributes to the achievement of Good Manufacturing Practice (GMP) and regulatory compliance criteria. 40
In a noteworthy instance, Bannigan et al. underscore the potential of machine learning (ML) technologies within the pharmaceutical field, highlighting ML's capacity to expedite the progress of drug delivery technologies. Despite challenges associated with neural network models, tree-based models like LGBM exhibit promise in mitigating the time and costs involved in the development of long-acting injectable (LAI) formulations. 41 Computational pharmaceutics, which includes machine learning and multiscale simulations, has altered traditional trial-and-error approaches in the realm of drug formulation for ocular, transdermal, pulmonary, and mucosal delivery systems. Recent advancements in molecular simulations, mathematical modelling, and pharmacokinetic/pharmacodynamic (PK/PD) modelling help to speed product development. In silico modeling and simulations provide detailed insights, facilitating a rational approach to formulation design and overcoming data challenges through interdisciplinary collaborations, particularly in the era of Pharma 4.0. 42, 43
AI for Pharmacokinetics and Pharmacodynamics
The medication development process is complicated, including stages such as drug discovery, preclinical investigations, clinical trials, and regulatory approval. Pharmacokinetics and pharmacodynamics, which determine the best dose, delivery method, and safety of a medicine within the body, are critical to this process. Traditional experimental approaches for evaluating these factors may be time-consuming and costly, and they frequently fail to provide reliable forecasts of therapeutic effectiveness and safety.44 Historically, pharmacokinetics and pharmacodynamics research depended on experimental approaches such as animal studies and human clinical trials, which presented issues such as ethical problems, sample size constraints, and inter-individual variability. 45 To overcome these challenges, computer models and artificial intelligence (AI) technologies have developed as viable tools for forecasting drug pharmacokinetics and pharmacodynamics, providing a faster, more cost-effective, and accurate approach. 46
AI has shown significant promise in the disciplines of pharmacokinetics, pharmacodynamics, and drug discovery. AI has proved useful in predicting and optimizing medication pharmacokinetics and pharmacodynamics as robust computing and machine learning methods have evolved. Despite limitations like as big datasets and data dependability, AI shows potential for furthering PKPD research and its influence on therapeutics. 47
Figure 1
Role of AI in Pharmacokinetics and Pharmacodynamics studies: AI is crucial in studying how medicines work in the body (PKPD). This involves complex calculations for drug absorption, distribution, metabolism, and excretion. AI accelerates these processes, preventing errors and delivering quicker, cost-effective, and accurate results. It simplifies data into graphs, aiding issue identification. Additionally, it reduces animal testing by calculating impacts, minimizing animals needed for trials.
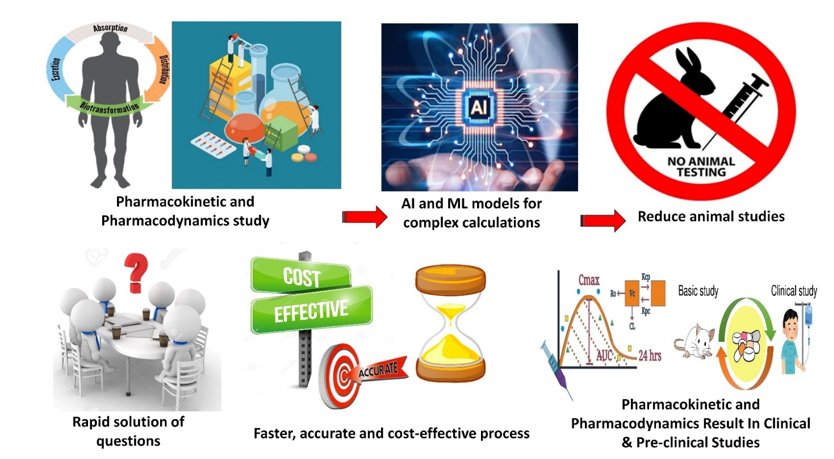
Methods using artificial intelligence to predict pharmacokinetic parameters
Machine learning and deep learning approaches are commonly employed in pharmacokinetic parameter prediction. ML approaches such as the Bayesian model, random forest, support vector machine, artificial neural network, and decision tree are used to predict drug absorption, distribution, metabolism, and excretion (ADME) properties. 48 Popular deep learning algorithms for computing pharmacokinetic parameters such as drug absorption, bioavailability, clearance, volume of distribution, and half-life include convolutional neural networks, long short-term memory, and recurrent neural networks. 49 Additionally, the quantitative structure-activity relationship (QSAR), a computational technique based on a molecule's chemical structure, is used to predict pharmaceutical ADME properties such as solubility, permeability, and metabolism. 50
AI-based computational method for PBPK
Physiologically Based Pharmacokinetic (PBPK) models, which are often used to simulate drug distribution and elimination inside the body, are complex and need a large amount of data and computer resources. AI-driven techniques to developing PBPK models aim to reduce dependency on animal research and human clinical trials. These techniques use machine learning algorithms to discover critical features and adjust model parameters, reducing the need for large amounts of data and computer power that has historically been associated with PBPK modelling. 51, 52
Prediction of drug release and absorption parameters
By analysing data from diverse drug delivery methods, AI-based models correctly forecast drug release and absorption characteristics. These models assess the rate and extent of drug release over time by taking into account parameters such as the drug's physicochemical qualities, formulation features, and the delivery system's release mechanism. 53 They also forecast drug release kinetics from various administration modalities, including as oral pills, transdermal patches, and inhalers. 54
Prediction of metabolism and excretion parameters
Models based on artificial intelligence evaluate drug metabolism and excretion parameters, offering insights into pharmaceutical pharmacokinetics. These models look at the molecular structure and physicochemical properties of medications to predict drug metabolism. AI models discover structural traits associated with specific metabolic transformations, compute enzyme kinetics, predict drug metabolic fate, and analyse possible influences on medication clearance and effectiveness by training on big datasets. To anticipate clearance rates and interactions with transporters involved in absorption, distribution, metabolism, and excretion processes, AI systems analyse drug physicochemical features.55, 56 This plethora of data assists in understanding drug disposition, optimising formulations, forecasting potential medication interactions, and prioritising drug candidates in order to make the drug development process more efficient.
AI in Disease Diagnosis
Ensuring a thoughtful treatment design and the well-being of patients hinges on the pivotal aspect of disease analysis. Human-generated inaccuracies pose challenges to precise diagnosis, and the misinterpretation of information compounds the complexity of this task. AI emerges as a versatile solution, instilling confidence in accuracy and efficiency. A comprehensive literature survey reveals diverse technologies and methodologies applied for disease diagnosis. The evolving human population and varied environmental manifestations continuously drive the escalating demand for the healthcare system. 57
Despite vulnerabilities, contradictions, and non-analyzing incongruities, recent evidence suggests the development of new methods to delineate their applicability, portraying previously unexplored scenarios. Categorizing patients based on disease severity underscores the importance of AI in the diagnostic process. Diagnosis, defined as the state designated upon pre-existing problems, emphasizes the importance of maintaining comprehensive health report forms for each patient. This practice aids in collecting reviews obtained through examinations and testing, enabling timely and tailored healthcare needs. 58
Clinician-driven analysis is subjective and may vary, highlighting the need for diagnostic strategies that focus on early disease prediction. AI assumes a crucial role in recognizing and ascertaining the early predictive stage of diseases, particularly in cancer and dementia cases. Current technological practices involve deep learning, neural networking, and algorithms for data identification, extraction, and processing. Notably, AI's influence is significant in diseases such as cancer and dementia, where unbiased algorithms, backed by relevant datasets, contribute to accurate predictions. 59
Deep learning associations adapt to evolutionary changes and enhance predictions, whereas unsupervised learning aids in hepatitis diagnosis. Although larger datasets and more diverse inputs improve AI applicability, the results can be difficult to understand. Deep learning applications in diagnostics include dermatological condition categorization and atrial fibrillation identification. Cross-validation is a technique for estimating algorithms that involves random splitting into different sets. The efficacy of AI is judged by its accuracy, sensitivity, and specificity, with these three critical elements emphasised in its typical assessments. 60
Following a review of the literature, clinical aspects overseeing deep learning networks and neural pathways, employing support vector machines, nearest neighbours, random forests, decision trees, logistic regression, naive Bayes, discriminant analysis, and convolutional neural networks, can produce more comprehensive results. 61 Algorithm-based performance analysis may be performed, taking into consideration aspects such as origin, sample size, and the amount of features in training and testing samples. The combination of decision trees and reasoning was applied for the detection of liver illnesses.
Numerous research on predictive modelling have been conducted, most notably in the prediction of early Parkinson's disease. 62 In the field of lung disorders, a rib segmentation algorithm based on chest X-ray pictures was developed to solve the limitations of standard rib-wise segmentation approaches. The algorithm achieves excellent performance, notably in rib segmentation, thanks to unpaired sample augmentation and a multi-scale network, and has potential applications in identifying lung cancer and other pulmonary disorders. 63 In recent study, algorithms and machine learning have been used to identify and classify cardiac arrhythmias by interpreting ECG information. 64 Another study used an optimisation genetic algorithm (GA) and a support vector machine (SVM) classifier to efficiently classify and diagnose TB. 65
AI in Digital Therapy
AI has the capacity to create meaningful connections within raw datasheets, allowing it to be used in illness diagnosis, treatment, and mitigation. Various innovative methodologies used for computational understanding in this expanding subject have the potential to be applied across a wide range of medical science fields. For addressing complicated clinical difficulties necessitates considerable knowledge acquisition, analysis, and application. The progress of medical AI aids doctors in the resolution of complex clinical situations. Artificial neural networks evolutionary computational models, fuzzy expert systems, and hybrid intelligent systems are all useful tools for healthcare workers when it comes to storing and processing data. 66, 67
The ANN operates on biological nervous system principles, with a network of linked computer processors known as neurons capable of parallel calculations for data processing. A binary threshold function was used to produce the first artificial neuron. 68 The multilayer feed-forward perceptron is a frequently used model that has three layers: an input layer, a middle layer, and an output layer. Each neuron is linked together via connections with numerical weights. 62
Paul Werbos presented "Backpropagation learning," a unique approach with a well-suited learning algorithm, in 1974. The artificial neural network (ANN) has found applications in image analysis, data interpretation, and waveform analysis. 69 Fuzzy logic, a branch of reasoning and inference, recognises and uses real-world occurrences by applying a continuous range of membership values ranging from 0 to 1, indicating false and true. Fuzzy controllers have been used in the operating room to give vasodilators and anaesthetics. 70
The survival of the fittest is emphasised in this evolutionary computing approach, which mimics the natural evolution process. The genetic algorithm, a popular method within this paradigm, investigates a large number of random solutions to a given issue and finally finds the best answer by rejecting inferior possibilities. AI is also important in the context of radiation. A new technical innovation, automated treatment planning, has shown to be quite beneficial in radiation treatment planning. This automated technique significantly improves plan quality, consistency, and error rates. There are three types of treatment workflows: automated rule implementation, logic modelling based on past clinical information, and multi-criteria optimisation.71
Clinical recommendations can be implemented using a simple computer programme with predetermined structures, allowing for the efficient examination of patient anatomy and physiology. This automated treatment planning system is capable of simulating the reasoning process used in manual treatment planning.72 Three-dimensional dose distribution and spatial dosage models, in particular, show promising accuracy. Radiomics, which makes use of multiple imaging biomarkers, gives complete information on tumours. This approach can be used to predict results and quantify toxicity in individual radiation treatment patients. 73
Limitations of AI
Despite the advantages offered by AI-based models, several limitations need careful consideration. These challenges, including the requirement for extensive datasets, potential biases, and interpretability issues, emphasize the importance of integrating AI with traditional experimental methods to ensure drug safety and efficacy. Here are the key limitations:
Limited availability of data
AI models heavily rely on substantial datasets for accurate predictions. However, limitations in data availability, especially for rare diseases or specific populations, can result in less accurate or biased predictions. Issues like incomplete representation of demographics in training datasets can lead to biased results. The quality and representativeness of data used for model development must be carefully considered.
Biases in data
The effectiveness of AI models is contingent on unbiased and complete training data. If biases or incompleteness exist in the data, predictions may be skewed. In clinical trials, homogeneity in patient populations can present problems, compromising the model's ability to predict drug efficacy accurately. Ensuring representative, trustworthy, and unbiased data is crucial for AI model development. 74
Inability to incorporate new data
Once trained, updating AI models with new data can be challenging, particularly in drug development where constant emergence of new information is common. Updating models requires significant time and resources, and failure to keep models current can result in inaccurate predictions and flawed decision-making. Strategies for model updates should be carefully designed.
Lack of transparency
AI models, often regarded as "black boxes" due to their complex algorithms, lack transparency in explaining how predictions are reached. This opacity poses challenges in obtaining regulatory approval, as it becomes difficult to demonstrate the accuracy and reliability of the model's predictions. Additionally, the lack of transparency may lead to a lack of trust in predictions, particularly when they conflict with the expectations of clinicians or researchers. 75
Constrained capability in addressing Variability
AI models, trained on extensive datasets, might encounter challenges in precisely forecasting drug responses for individuals who deviate significantly from the norm. This issue is particularly noteworthy for drugs exhibiting diverse responses in various patients, where substantial variability is a key factor. 76
Interpretation of results
The complexity of AI models can lead to outputs that are challenging to interpret, even for experts. The lack of clear explanations for predictions poses challenges for clinicians and researchers in understanding and translating results into actionable insights. Improved interpretability and explainability of AI models are essential for effective use. 77
Ethical considerations
Ethical concerns, such as patient privacy, data ownership, and consent issues, arise when using AI in drug development. The collection and use of sensitive health data demand careful attention to protect privacy and respect rights. Regulatory agencies play a crucial role in developing protocols and guidelines to address these ethical considerations. 78
Lack of clinical expertise
While AI can identify correlations, it may struggle to comprehend the intricacies and profound effects of specific parameters, especially in individualized patient therapies. The statistical nature of AI algorithms may limit their understanding of complex factors influencing treatment decisions, particularly in scenarios where personalized factors play a significant role. 79
In conclusion, recognizing and addressing these limitations is crucial for the responsible integration of AI in drug development, ensuring that its potential benefits align with ethical and regulatory standards. Advances in interpretability, data quality, and ongoing model updates are essential for overcoming these challenges.
Conclusion and Future Prospective
The integration of Artificial Intelligence (AI) is poised to usher in a transformative era for the pharmaceutical industry, particularly in drug discovery, development, and delivery. Virtual screening techniques, empowered by AI, will expedite the analysis of vast chemical libraries, accelerating the identification of therapeutic candidates and lead compounds. AI's capabilities extend to enabling precise medicine, categorizing patients, predicting therapy responses, and customizing medicines based on genomic, proteomic, and clinical data analysis.
In drug development, AI holds the promise of creating innovative compounds with target-binding characteristics through deep learning and generative models, thereby enhancing medication effectiveness and reducing adverse effects. Patient-specific dose formulations and safety assessments are areas where AI algorithms are anticipated to play pivotal roles, optimizing medicine compositions and improving treatment outcomes while predicting potential side effects and toxicity. AI-powered monitoring systems, wearable gadgets, and sensors will facilitate remote patient care, ensuring better medication adherence through personalized therapy recommendations. AI's influence extends to clinical trial design, patient selection, and recruitment, utilizing electronic health records and genetic profiles to identify appropriate patients, lower trial costs, and expedite approval processes. Moreover, AI's real-time monitoring and control capabilities are expected to optimize continuous manufacturing operations in pharmaceuticals, ensuring uniformity and efficiency. The potential of AI in regulatory decision-making is significant, as it analyzes large datasets to inform decisions, expediting medication approval and enhancing safety measures.
To summarise, AI is poised to transform medication delivery technology by providing targeted and personalized therapeutics. AI-based models for pharmacokinetics and pharmacodynamics provide benefits over traditional techniques, possibly eliminating the need for animal research and clinical trials. The incorporation of AI technology offers significant potential for speeding up drug development, improving patient outcomes, and catapulting the pharmaceutical sector into the next evolutionary stage, from 4.0 to 5.0. While challenges like as data quality, regulatory frameworks, and ethical issues must be addressed, continuous breakthroughs and partnerships hold significant promise for a paradigm change in pharmaceutical procedures.